Sequence to sequence ( Seq2Seq )の技術を紹介します。
機械学習界隈ではブレイクスルーな技術としてGANやVAEが話題となりました。今回解説するSeq2Seqもブレイクスルーといわれる技術の1つです。
近年、劇的に日本語の翻訳技術が向上しました。その技術の背景にはSeq2Seqが使われています。Seq2Seqの得意分野は翻訳もそうですが、自動字幕技術、あるいはチャットボットなどのQuestions answeringの技術であり、連続値などのシーケンシャルなデータの取扱が得意です。
Seq2SeqはGoogleにより2014年に発表されました。Googleは2015年頃の論文でSpeech recognition[1]やビデオ字幕[2]で劇的に精度が向上したと発表しています。
今回はそんなSeq2Seqの技術を解説し、最後にPyTorchでの実装例を紹介します。
Sequence to Sequence の構造
Seq2Seqは2つのパートに別れます。EncoderとDecoderです。形的にはAutoEncoder(例えばVAE)に少し似ています。ただ、中の実装方式がまるで違います。
Seq2SeqはRNNを利用しているため時系列データに強いという所が特徴です。 そのため翻訳や音声認識の分野で力を発揮しているのです。
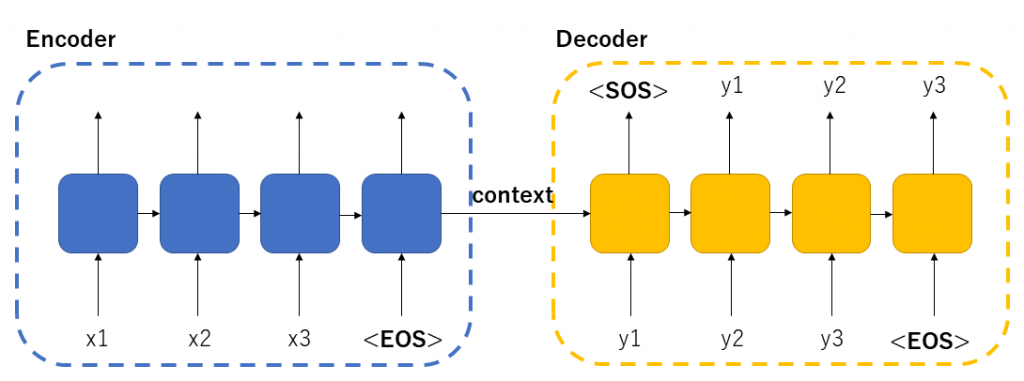
Encoder、ならびにDecoderの内部では横矢印でデータをそれぞれ前段のデータを数珠つなぎに渡しています。RNNの特徴で、学習時に前段の特徴をわたすことにより時系列データに強くなるのです。
Seq2Seqを理解するに辺りRNNの概念の理解が必要です。本ブログのRNNの記事の概念の部分を読んで把握しておいてください。
それではEncoderとDecoderを実際にどういう仕組みや役割を担っているのか見ていきます。
EncoderとDecoder
Seq2Seqで作られた翻訳エンジンを例にEncoderとDecoderの役割を解説します。翻訳とは「こいつは犬。」という入力に対し、「 This is a dog.」と解答が得ることと定義します。
Encoder部は、日本語で「こいつは犬。」を入力するパートになります。
Decoder部は 、「This is a dog.」という入力、並びにEncoderから出る横矢印の隠れ層のデータ(Context)を受け取って、入力と同じ「This is a dog.」という出力を得るパートです。やや複雑ですが、下の図を見て、理解をしてください。
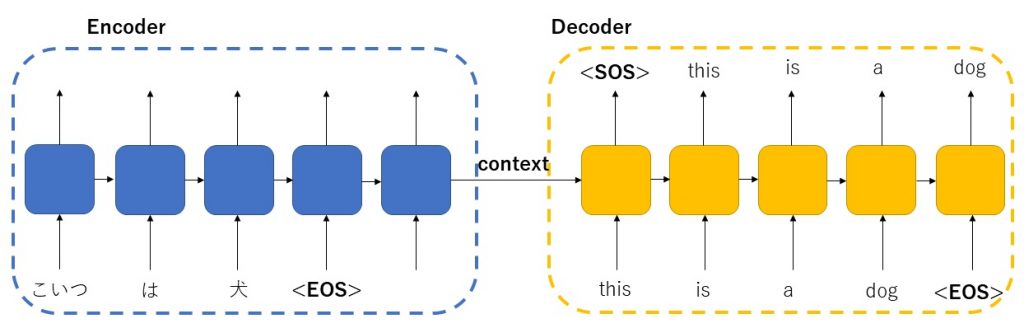
上の図を見ると面白いことに気づきます。「こいつ は 犬」と3つ単語に対し、「This is a dog」と4つの単語が出力されます。 Seq2Seqの特徴ですが入力数と出力数が一致していなくてもいいのがユニークなところです。上の図の説明ではEncoder&Decoderともに5つの素子で構成しています。実際には最大の文章の単語数以上の素子を用意しておくことになります。
Decoder部分に注目してみます。入力値として英語の答えを入力し、出力値として、一弾ずつずれて同じものを期待するように設計しています。
<SOS>,< EOS>はStart of string, End of stringの略ですが、学習時にはこれをつけて学習していきます。これを伝えることで、文の始まりと終わりを伝えるのです。
Encoderの出力値については捨てられます。ただし、使うことに依り精度を上げる方法(+Attention法)もあります。アルゴリズムの選択により使ったり使わなかったりするのです。
さて、DecoderとEncoderをつなげるのは、Encoderで学習したHiddenベクトル(Context)となります。
以上が簡単な説明になります。世界を圧巻したSeq2Seqですが意外と簡単な構造をしていたんだな、と思うと思います。それではいよいよPyTorchで実装していきます。
実装
今回はPytorchの公式のSeq2Seqを参考にソースコード解説をします。本家はやや説明に冗長なコードがありますので、Seq2seqを理解するためだけのコードにしました。
下準備(学習データ)
学習には次のファイルを使いましょう。
実装する上では学習データを用意しないと学習できません。残念ながらPyTorchでは標準で日本語データサポートしていないので、他社サイトからデータを取得します。
今回はこちらのサイトからデータを取得しました。流れとしては、そして日本語と英語の2つのファイルに分けました。日本語は英語のようにスペースで分けられていないので、分かち書き(形態素解析)によって分割しました。日本語には半角全角といった表記ゆれもあるのでそうしたものを正規化処理します。具体的にはそれぞれMecab, unicodedata.normalizeなどを使うのですがその辺りは今回のseq2seq技術と全く関係ないのでここでは説明しません。
ファイルの1行1行の日本語と英語が1対1対応しています。
他の言語でもテストしてみたい場合は、こうした学習データを作り、独自に学習させてみてください。
また、import文と、言語の処理クラスLangをインポートします。Lang クラスではwordをindex化したりするクラスです。
import random import torch import torch.nn as nn from torch import optim import torch.nn.functional as F SOS_token = 0 EOS_token = 1 device = "cuda" # torch.device("cuda" if torch.cuda.is_available() else "cpu") class Lang: def __init__( self, filename ): self.filename = filename self.word2index = {} self.word2count = {} self.sentences = [] self.index2word = { 0: "SOS", 1: "EOS" } self.n_words = 2 # Count SOS and EOS with open( self.filename ) as fd: for i, line in enumerate( fd.readlines() ): line = line.strip() self.sentences.append( line ) self.allow_list = [ True ] * len( self.sentences ) self.target_sentences = self.sentences[ :: ] def get_sentences( self ): return self.sentences[ :: ] def get_sentence( self, index ): return self.sentences[ index ] def choice( self ): while True: index = random.randint( 0, len( self.allow_list ) - 1 ) if self.allow_list[ index ]: break return self.sentences[ index ], index def get_allow_list( self, max_length ): allow_list = [] for sentence in self.sentences: if len( sentence.split() ) < max_length: allow_list.append( True ) else: allow_list.append( False ) return allow_list def load_file( self, allow_list = [] ): if allow_list: self.allow_list = [x and y for (x,y) in zip( self.allow_list, allow_list ) ] self.target_sentences = [] for i, sentence in enumerate( self.sentences ): if self.allow_list[ i ]: self.addSentence( sentence ) self.target_sentences.append( sentence ) def addSentence( self, sentence ): for word in sentence.split(): self.addWord(word) def addWord( self, word ): if word not in self.word2index: self.word2index[ word ] = self.n_words self.word2count[ word ] = 1 self.index2word[ self.n_words ] = word self.n_words += 1 else: self.word2count[word] += 1 def tensorFromSentence( lang, sentence ): indexes = [ lang.word2index[ word ] for word in sentence.split(' ') ] indexes.append( EOS_token ) return torch.tensor( indexes, dtype=torch.long ).to( device ).view(-1, 1) def tensorsFromPair( input_lang, output_lang ): input_sentence, index = input_lang.choice() output_sentence = output_lang.get_sentence( index ) input_tensor = tensorFromSentence( input_lang, input_sentence ) output_tensor = tensorFromSentence( output_lang, output_sentence ) return (input_tensor, output_tensor)
Encoder
Encoderの実装は次のとおりです。
class Encoder( nn.Module ): def __init__( self, input_size, embedding_size, hidden_size ): super().__init__() self.hidden_size = hidden_size # 単語をベクトル化する。1単語はembedding_sie次元のベクトルとなる self.embedding = nn.Embedding( input_size, embedding_size ) # GRUに依る実装. self.gru = nn.GRU( embedding_size, hidden_size ) def initHidden( self ): return torch.zeros( 1, 1, self.hidden_size ).to( device ) def forward( self, _input, hidden ): # 単語のベクトル化 embedded = self.embedding( _input ).view( 1, 1, -1 ) # ベクトル化したデータをGRUに噛ませる。通常のSeq2Seqでは出力outは使われることはない。 # ただしSeq2Seq + Attentionをする場合にはoutの値を使うことになるので、リターンする out, new_hidden = self.gru( embedded, hidden ) return out, new_hidden
Encoderでは文字列をEmbeddingします。Embeddingとは単語をベクトル化することです。例えばDogを5次元にEmbeddingするとするとDog–>[0.9, 0.5 0.4, 0.7, 0.1] のようにすることを意味します。
Embeddingは実はword2vecを用いたほうが精度が良いようですが、とりあえず今はSeq2Seqの実装とは関係ないので標準のライブラリを使います。精度を上げたい人はこの部分を改良してみても面白いでしょう。
Embeddingされた単語をRNNのネットワークに入れるのですが、PyTorchではRNN系として、nn.LTSM, nn.RNN, nn.GRUというものが既にあり、自分で数珠つなぎのRNN素子を定義してネットワークを書く必要はありません。RNNモジュールの入力次元はEmbeddingする次元になります。
実装ではGRUかLSTMで世の中では良く取り沙汰されています。今回はPyTorchの公式ドキュメントでGRUであったのと、日本語のドキュメントサイトでLSTMが多かったのでGRUで説明します。LSTMで実装したいなどあれば適時、ソースコードを書き換えてみてください。(nn.LSTMは出力がGRUとことなるので注意が必要です。view関数などを使って出力数を変更する必要もあります。)
Decoder
Decoderの実装は次のとおりです。
class Decoder( nn.Module ): def __init__( self, hidden_size, embedding_size, output_size ): super().__init__() self.hidden_size = hidden_size # 単語をベクトル化する。1単語はembedding_sie次元のベクトルとなる self.embedding = nn.Embedding( output_size, embedding_size ) # GRUによる実装(RNN素子の一種) self.gru = nn.GRU( embedding_size, hidden_size ) # 全結合して1層のネットワークにする self.linear = nn.Linear( hidden_size, output_size ) # softmaxのLogバージョン。dim=1で行方向を確率変換する(dim=0で列方向となる) self.softmax = nn.LogSoftmax( dim = 1 ) def forward( self, _input, hidden ): # 単語のベクトル化。GRUの入力に合わせ三次元テンソルにして渡す。 embedded = self.embedding( _input ).view( 1, 1, -1 ) # relu活性化関数に突っ込む( 3次元のテンソル) relu_embedded = F.relu( embedded ) # GRU関数( 入力は3次元のテンソル ) gru_output, hidden = self.gru( relu_embedded, hidden ) # softmax関数の適用。outputは3次元のテンソルなので2次元のテンソルを渡す result = self.softmax( self.linear( gru_output[ 0 ] ) ) return result, hidden def initHidden( self ): return torch.zeros( 1, 1, self.hidden_size ).to( device )
ほとんどEncoderと一緒です。ただRelu活性関数を適用したり、最後に全結合してSoftmax関数を噛ませているところに違いがあります。また、Decoderでは入力値として、前段のEncoderからのHiddenベクトルをもらうところが違いがあります。
メイン関数
EncoderとDecoderを用いたメイン関数は次のとおりです。
def main(): n_iters = 75000 learning_rate = 0.01 * 0.8 embedding_size = 256 hidden_size = 256 max_length = 30 input_lang = Lang( 'jpn.txt' ) output_lang = Lang( 'eng.txt') # 英単語数がmax_lengthより多い場合は計算しない。(時間がかかるため。) allow_list = [x and y for (x,y) in zip( input_lang.get_allow_list( max_length ), output_lang.get_allow_list( max_length ) ) ] # allow_listに従って、英語、日本語のファイルをロードする input_lang.load_file( allow_list ) output_lang.load_file( allow_list ) # Encoder & Decoderの定義 encoder = Encoder( input_lang.n_words, embedding_size, hidden_size ).to( device ) decoder = Decoder( hidden_size, embedding_size, output_lang.n_words ).to( device ) # Optimizerの設定 encoder_optimizer = optim.SGD( encoder.parameters(), lr=learning_rate ) decoder_optimizer = optim.SGD( decoder.parameters(), lr=learning_rate ) # 学習用のペアデータの作成. He is a dog, 彼は犬だ みたいなペアをエポック数分用意する training_pairs = [ tensorsFromPair( input_lang, output_lang ) for i in range( n_iters ) ] # LOSS関数 criterion = nn.NLLLoss() for epoch in range( 1, n_iters + 1): # 学習用のペア単語の取り出し。 input_tensor, output_tensor = training_pairs[ epoch - 1 ] #初期化 encoder_hidden = encoder.initHidden() encoder_optimizer.zero_grad() decoder_optimizer.zero_grad() input_length = input_tensor.size(0) output_length = output_tensor.size(0) # Encoder phese for i in range( input_length ): encoder_output, encoder_hidden = encoder( input_tensor[ i ], encoder_hidden ) # Decoder phese loss = 0 decoder_input = torch.tensor( [ [ SOS_token ] ] ).to( device ) decoder_hidden = encoder_hidden for i in range( output_length ): decoder_output, decoder_hidden = decoder( decoder_input, decoder_hidden ) # 次の入力野取り出し decoder_input = output_tensor[ i ] # 学習では一定の確率(ここでは50%)で、自身が前に出力した単語を次の入力とする。 if random.random() < 0.5: # 確率が最も高い単語を抽出 topv, topi = decoder_output.topk( 1 ) # 確率が一番高かった単語を次段の入力とする decoder_input = topi.squeeze().detach() # Loss関数 loss += criterion( decoder_output, output_tensor[ i ] ) # EOSに当たった場合は終わる。 if decoder_input.item() == EOS_token: break loss.backward() encoder_optimizer.step() decoder_optimizer.step() # 進捗状況の表示 if epoch % 50 == 0: print( "[epoch num %d (%d)] [ loss: %f]" % ( epoch, n_iters, loss.item() / output_length ) )
流れに関してはコメントアウトにて記載しました。
実装をする上で、言語を管理するLangクラスを定義しました。内容はSeq2seqの技術と関係ないので割愛しますが、Langクラスについては、Githubにあるmain.pyを参考にしてください。
評価関数
学習がきちんとできたか、実際確かめる評価関数は次のとおりです。
def evaluate( sentence, max_length ): input_lang = Lang( 'jpn.txt') output_lang = Lang( 'eng.txt' ) allow_list = [x and y for (x,y) in zip( input_lang.get_allow_list( max_length ), output_lang.get_allow_list( max_length ) ) ] input_lang.load_file( allow_list ) output_lang.load_file( allow_list ) hidden_size = 256 embedding_size =256 encoder = Encoder( input_lang.n_words, embedding_size, hidden_size ).to( device ) decoder = Decoder( hidden_size, embedding_size, output_lang.n_words ).to( device ) enfile = "OUTPUT_FILE_FROM_ENCODER" defile = "OUTPUT_FILE_FROM_DECODER" encoder.load_state_dict( torch.load( enfile ) ) decoder.load_state_dict( torch.load( defile ) ) with torch.no_grad(): input_tensor = tensorFromSentence(input_lang, sentence) input_length = input_tensor.size()[0] encoder_hidden = encoder.initHidden() for ei in range(input_length): encoder_output, encoder_hidden = encoder(input_tensor[ei], encoder_hidden) decoder_input = torch.tensor([[SOS_token]], device=device) # SOS decoder_hidden = encoder_hidden decoded_words = [] decoder_attentions = torch.zeros(max_length, max_length) for di in range(max_length): decoder_output, decoder_hidden = decoder( decoder_input, decoder_hidden ) topv, topi = decoder_output.data.topk(1) if topi.item() == EOS_token: decoded_words.append('<EOS>') break else: decoded_words.append(output_lang.index2word[topi.item()]) decoder_input = topi.squeeze().detach() return decoded_words, decoder_attentions[:di + 1] if __name__ == '__main__': import MeCab import unicodedata wakati = MeCab.Tagger("-Owakati") sentence = 'とても悲しいです.' sentence = unicodedata.normalize( "NFKC", sentence.strip() ) a=wakati.parse( sentence.strip() ).split() ret =" ".join( a ) print(evaluate( ret, 30 ) )
enfile, defileは学習時したデータのPATHを記載します。
注意ですが、hidden_sizeとmax_lengthは学習時と同じ値を使うようにします。
encoder.load_state_dict(torch.load(FILE_PATH))により、実際に学習したデータをロードしています。
結果
60をmax_length、epoch数を150,000として学習させた結果は次の通りです。学習には40分程かかります。(GPUを利用した場合)
海外 に 旅行 に 行き たい .
([‘i’, ‘want’, ‘to’, ‘go’, ‘to’, ‘the’, ‘trip’, ‘.’, ”],
この 映画 は 面白い です か ?
([‘how’, ‘movie’, ‘is’, ‘this’, ‘movie’, ‘?’, ”]
これ は 料理 です .
([‘this’, ‘is’, ‘a’, ‘good’, ‘cook’, ‘.’, ”]
この 机 は 私 の 一番 の お気に入り です .
([‘this’, ‘is’, ‘is’, ‘most’, ‘of’, ‘mine’, ‘.’, ”],
彼 は とても いい 人 です .
([‘he’, ‘is’, ‘a’, ‘good’, ‘person’, ‘.’, ”]
さて、この結果考察をどう思うでしょうか。たかだか40分の学習時間、かつ愚直なデータでこの精度までいきました。正直驚きです。機会翻訳の分野で一生懸命やっていた人は更に驚くのではないでしょうか?
色々と問題があるものの、学習数を多くする、登録単語数を増やす、同じ学習データを何度も流し込む、など色々なアプローチで、明らかな文法ミスに対してペナルティを高くするなどしていくと劇的な変化が見られるのではないかと思います。又学習速度に関してもバッチ化することで高速化が見込めます。
興味がある人は是非トライしてみてください。多くのデータサイエンティスト、或いは機械学習のエンジニアがやっていく作業がこういう泥臭い作業になっていきます。
最後に
今回のソースコードはgithubにあげてあります。[Seq2Seq Github]
Githubでは、本稿では取り上げていない+attention法も実装してあります。
Attention法とは長文になると精度が悪くなるという弱点を補強したアルゴリズムです。そのため、短文で10単語くらいの簡単なものに関しては精度が劇的に上がることはありません。Attentionに関して、詳しくは次のTransformerもご参照ください。トランスフォーマーは、Seq2Seqの問題点を解決し、2022年現状で最も高い精度を誇る機械学習方法となっています。(GPT-3, BERTなどの元になっているやつです)
Seq2Seqでは、EncoderやDecoderを多層にしたり、Bidirectionalにしたり、GRUの代わりにLSTMを使ったり、というような色々な工夫があります。それぞれの方法でどれが良いかについてはここで述べられています[3]
実際に実装してみて、どれが精度が良いのかなど試してみると面白いと思いでしょう。
参考文献
- https://www.isca-speech.org/archive/Interspeech_2017/pdfs/0233.PDF
- https://arxiv.org/pdf/1505.00487.pdf
- https://arxiv.org/pdf/1908.04332.pdf
- https://towardsdatascience.com/understanding-encoder-decoder-sequence-to-sequence-model-679e04af4346
- https://pytorch.org/tutorials/intermediate/seq2seq_translation_tutorial.html